Floriane Montanari
Bayer AG, Berlin, Germany
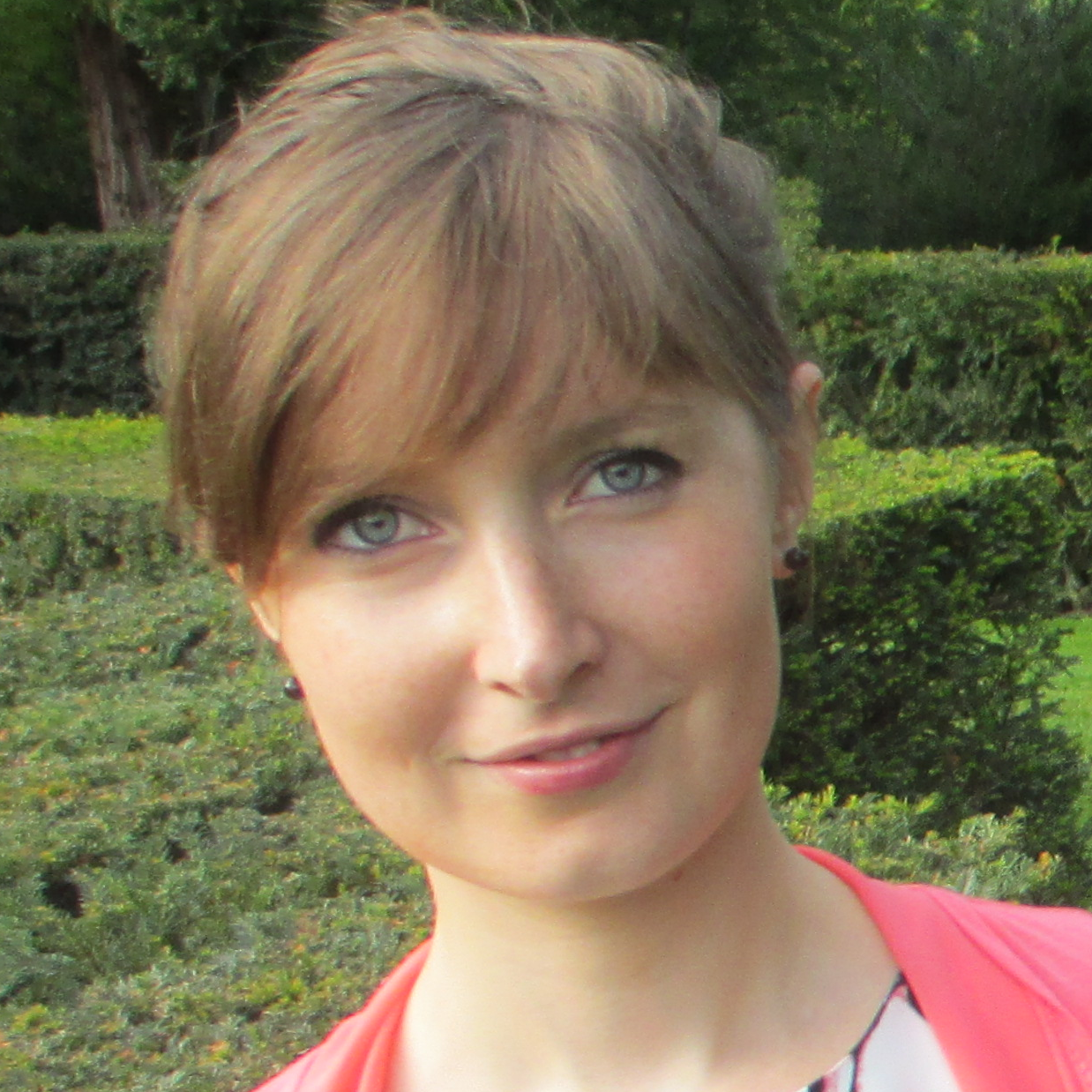
Biography: I am a research scientist with years of experience in cheminformatics. I have a passion for useful machine learning approaches applied at all stages of the drug discovery pipeline. Since May 2017 I am working at Bayer, where I have been lucky enough to improve the daily routine of chemists company-wide by productionizing my work on deep learning for ADMET properties. I am the co-author of more than fifteen scientific publications and in 2016 I received a PhD from the University of Vienna, with a thesis focusing on ABC-transporters inhibition and best practices in model validation. I enjoy participating in competitions and in 2014 I submitted the best predictive model in the Teach-Discover-Treat challenge on Malaria HTS prediction.
Deep learning for computational chemistry: compound representation, ADMET profiles and automatic optimization
One of the main challenges in small molecule drug discovery is efficiently finding novel chemical compounds with desirable properties. Such properties can be physico-chemical (like logD or solubility), pharmacokinetic (like permeability, clearance or metabolic stability) or pharmacodynamic (like biological activity on targets of interest).
Computational chemistry has since long been involved in the drug discovery process from hit selection to lead optimization. In silico methods allow for fast and cost-effective filtering steps before the chemical matter is even synthesized.
Here, we discuss how deep learning can be utilized for many different aspects of modeling in chemistry. In cheminformatics, the first step is to describe the chemical matter in a computer-readable way. We present two alternatives to the commonly applied circular fingerprints: graph convolutions on the molecular graph and a sequence-to-sequence autoencoder on SMILES notations. We then demonstrate how combining different ADMET endpoints together in one multitask deep learning model can boost the predictive performance compared to its single-task alternatives, especially on endpoints that are more difficult to model. Finally, combining our reversible encoding of the chemical space with improved predictive models and an optimization algorithm, we demonstrate how a query compound can be optimized with respect to multiple (predicted) molecular properties. We hope that our method will support medicinal chemists in accelerating and improving the lead optimization process by proposing synthesis ideas and handling multi-objective optimization.