Peter Horvath
Biological Research Centre of the Hungarian Academy of Sciences, Hungary
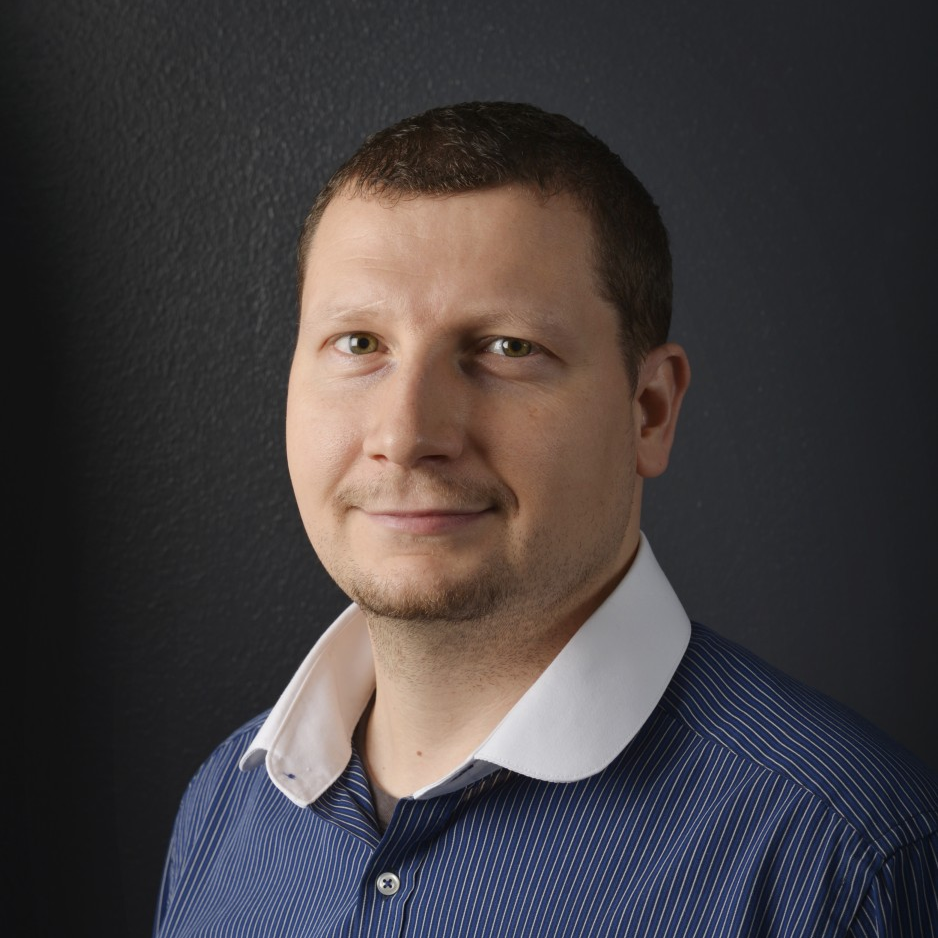
Biography: Peter Horvath (1980) is currently a director and group leader in the Biological Research Center of the Hungarian Academia of Sciences in Szeged and holds a Finnish Distinguished Professor (FiDiPro) Fellow position in the Institute for Molecular Medicine Finland (FIMM), Helsinki. He graduated as a software engineer and mathematician, and received his Ph.D. from INRIA and University of Nice, Sophia Antipois, France in satellite image analysis. Between 2007 and 2013 he was a senior scientist at the ETH Zurich, in the Light Microscopy Centre. Peter Horvath is interested in solving computational cell biology problems related to light microscopy and is involved in three main research fields; 2/3D biological image segmentation and tracking; development of microscopic image correction techniques; machine learning methods applied in high-throughput microscopy. He is the co-founder of the European Cell-based Assays Interest Group and the councillor of the Society of Biomolecular Imaging and Informatics.
Life beyond the pixels: machine learning and image analysis methods for HCS
In this talk I will give an overview of the computational steps in the analysis of a single cell-based high-content screen. First, I will present a novel microscopic image correction method designed to eliminate vignetting and uneven background effects which, left uncorrected, corrupt intensity-based measurements. I will discuss the Advanced Cell Classifier (ACC) (www.cellclassifier.org), a software tool capable of identifying cellular phenotypes based on features extracted from the image. It provides an interface for a user to efficiently train machine learning methods to predict various phenotypes. We developed the Suggest a Learner (SALT) toolbox, which selects the optimal machine learning algorithm and parameters for a particular classification problem. For cases where discrete cell-based decisions are not suitable, we propose a method to use multi-parametric regression to analyze continuous biological phenomena. Finally, to improve the learning speed and accuracy, we recently developed an active learning scheme which automatically selects the most informative cell samples.