Panče Panov
Jožef Stefan Institute, Slovenia
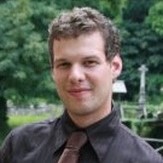
Biography: Panče Panov is a postdoctoral researcher at the Department of Knowledge Technologies, JSI. In addition, he is a part-time assistant professor at the Jožef Stefan International Postgraduate School in Ljubljana and the Faculty of information studies in Novo mesto. He completed his PhD in 2011 at the Jožef Stefan International Postgraduate School in Ljubljana on the topic of ontologies for data mining. His research interests are in the field of data mining and machine learning, as well as knowledge representation in different domains using ontologies. His current research is aimed towards further development of ontological models and resources for describing the objects and processes in data mining and machine learning. He has participated in several national Slovenian projects, the EU funded projects such as FP6 FET IQ, FP7 FET Open project MAESTRA and is also involved in the Human Brain Project. Currently, he is coordinating a national project named IMPERATRIX on the topic of reproducibility of experiments and reusability of research results in data analytics.
Improving the reproducibility of experiments and reusability of research outputs in complex data analysis
The advances in science are heavily based on the premise of the concept of a trusted discovery, provided that the performed research is done correctly, and reproducible by other scientists. In order to increase the reusability of research outputs, such as developed models and produced data, they should be Findable, Accessible, Interoperable and Reusable (FAIR principles). The main point of the FAIR is to ensure that research outputs are reusable and will actually be used by others, thus becoming more valuable. The research outputs that wish to fulfil the FAIR principles must be represented with a wide accepted machine-readable framework. Currently, a popular solution to data sharing that fulfils the FAIR requirements is the use of semantic web technologies and ontologies.
Complex data analysis methods, originating from machine learning and data mining, are increasingly being used in applications from various domains of science (e.g., life sciences, space research, etc). In order to provide reproducibility of experiments (e.g., executions of methods) and reuse of research outputs (e.g., predictive models), one needs to formally describe the entities involved in the process of analysis, and store them together with their descriptions (e.g., metadata) as a digital objects in a database like structure. Having a “semantically aware” stores of entities for complex data analytics enhanced with automatic reasoning capabilities would be beneficial for improving the reproducibility of experiments and reuse of research outputs. In this way, we would move closer to a FAIR data analysis process.
In this talk, I will show and discuss the recent advances in the domain that are aimed towards improving the reproducibility of experiments and reusability of research outputs in complex data analysis.