Noé Sturm
IMED Biotech Unit, AstraZeneca Göteborg, Sweden
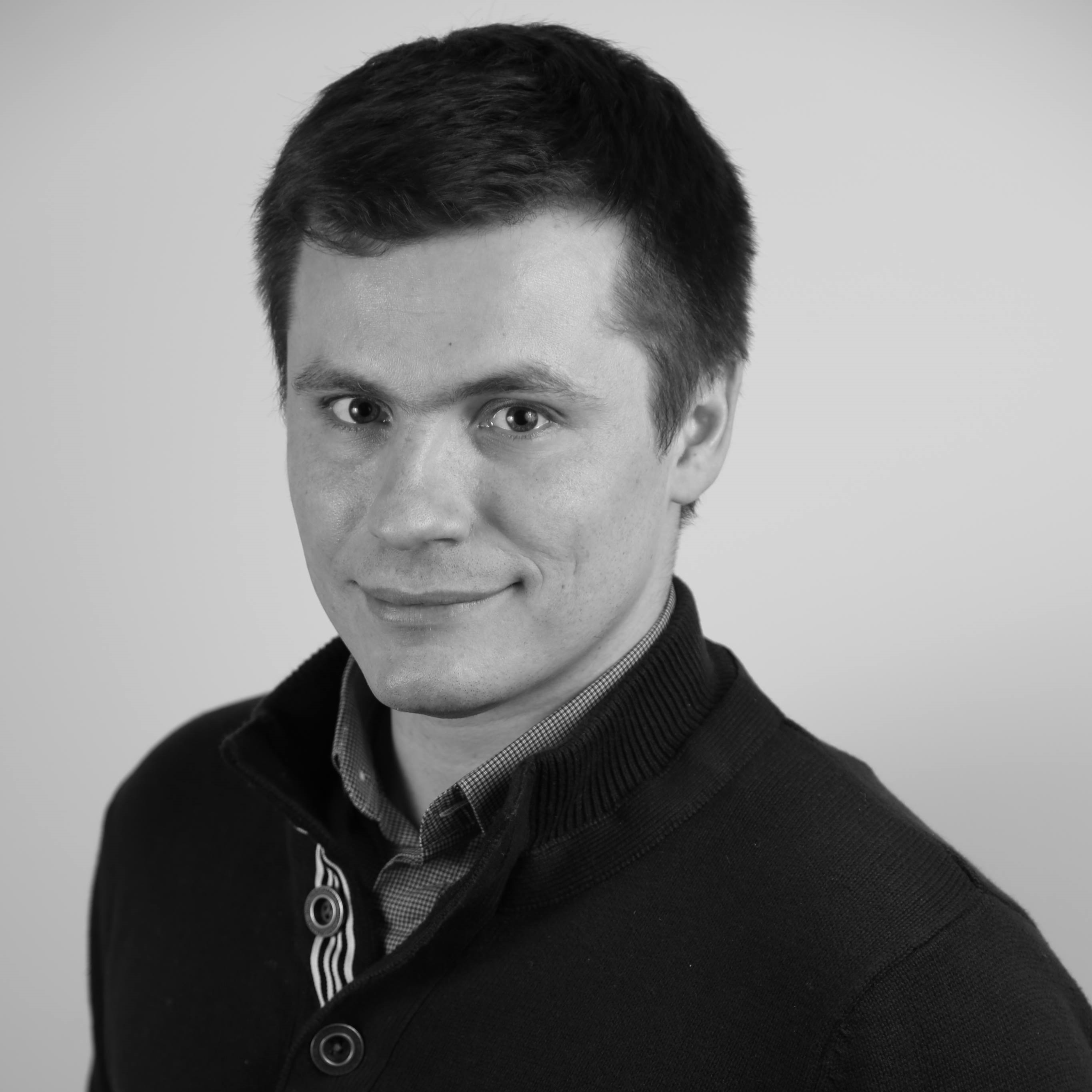
Biography: Noé received his PhD in chemistry with a specialization in cheminformatics at the University of Strasbourg, France and in partnership with Griffith University in Brisbane, Australia. During his PhD, he studied structural relationships between natural product biosynthetic enzymes and drug target proteins in the context of pharmacognosy. After a 1-year postdoc at the University of Modena and Reggio Emilia, Italy - where he worked on the development of allosteric inhibitor of EGFR with computer-aided methods – he joined Astrazeneca as part of the European project ExCAPE. His role was to apply state of the art machine learning technologies such as deep learning and matrix factorization to very large chemogenomics datasets to generate large scale bioactivity prediction models. Since January 1st, 2019, he holds an position in the Data Science and AI team at AstraZeneca and is working on drug-safety related topics.
Building Chemogenomics Models from a Large-Scale Public Dataset and Applying them to Industrial Datasets
ExCAPE was a European funded project aiming at harvesting the power of supercomputers to speed up drug discovery (http://excape-h2020.eu/). Thanks to the project team, we were given the amazing opportunity to build large-scale machine learning models for compound activity predictions from public databases and to apply them to industrial datasets. In this talk, I will present the process of collecting chemogenomics data from public resources to build a benchmark dataset. Subsequently, I will explain the process of building and evaluating the performance of models built with multi-task deep learning and matrix factorization algorithms. Ultimately, I will show how these models were applied to industrial datasets.