Larisa Soldatova
Goldsmiths, University of London, UK
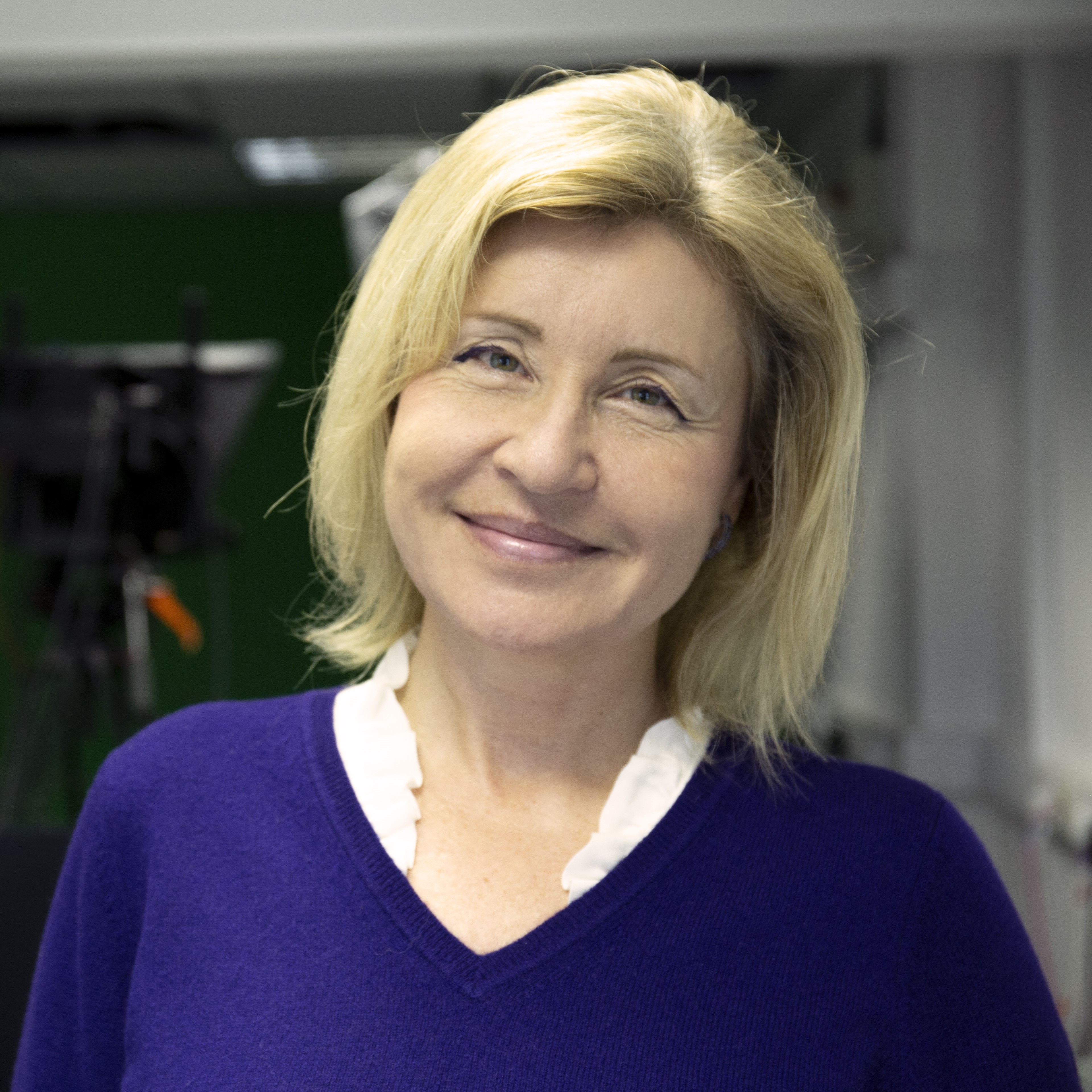
Biography: Dr Larisa Soldatova is Reader in Data Science in Goldsmiths, University of London and Director of online MSc Data Science programme. Larisa is an internationally recognised expert in knowledge representation, semantic technologies, data analytics and their application to the life sciences. She is involved in a number of international projects on the development of semantic standards, e.g. the Ontology for Biomedical investigations (OBI), the ontology for Data Mining, ML schema, SBOL (synthetic biology open language) – visual, laboratory protocols EXACT. Selected awards: BCS Machine Intelligence prize (2006), nomination for the World Technology Award (Software) (2006), RCUK Fellowship (2007-2012), Meta-QSAR grant (EPSRC) (2012-2014), AdaLab grant (EPSRC) (2014-2018), Big Mechanism Grant (DARPA) (2014-2018), ACTION on cancer grant (EPSRC) (2018-2022).
Meta-QSAR and Multi-Task QSAR Learning
Larisa will present the results of the meta-QSAR project funded by EPSRC (Engineering and Physical Sciences Research Council UK) (‘learning to learn how to design drugs’ EP/K030469/1, EP/K030582/1). Although almost every type of machine learning method has been applied to QSAR learning there is no agreed single best way of learning QSARs. The project team of researchers carried out the most comprehensive ever comparison of machine learning methods for QSAR learning: 18 regression methods, 6 molecular representations, applied to more than 2,700 QSAR problems. They then investigated the utility of algorithm selection for QSAR problems. They found that such a meta-learning approach outperformed the best individual QSAR learning method (i.e. random forests using a molecular fingerprint representation) by up to 13%, on average. It provides evidence for the general effectiveness of meta-learning over base-learning.
The meta-QSAR project team also employed multi-task learning (MTL) to exploit commonalities in drug targets and assays. They analysed over a thousands of assay provided by ChEMBL. They carried out feature-based and instance-based MTL to predict drug activities. In addition, they introduced a natural metric of evolutionary distance between drug targets as a measure of tasks relatedness. The results of MTL studies were compared with the results of a single task learning, a random forest as the best performing QSAR learner. The results are: instance-based MTL significantly outperformed both, feature-based MTL and the base learner. MTL was significantly improved by incorporating the evolutionary distance between targets.
The results of the meta-QSAR project have been made publicly available on OpenML platform.