Günter Klambauer
Johannes Kepler University Linz, Austria
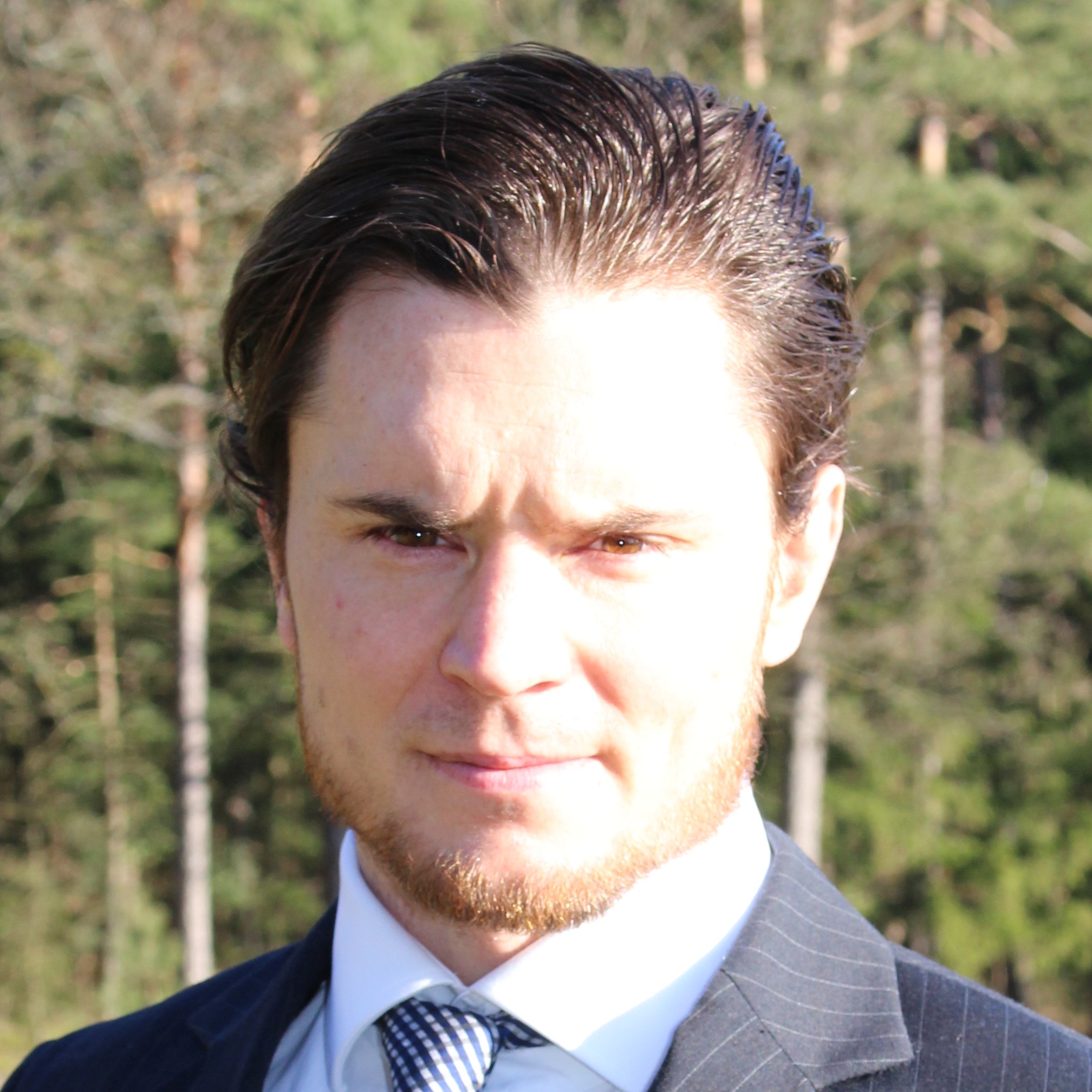
Biography: After studying Mathematics and Biology at the University of Vienna, Günter Klambauer started his research in bioinformatics and machine learning in 2010 at the Johannes Kepler University Linz, where he earned his PhD in 2014. His machine learning methods for genetics were awarded with the Austrian Life Science Award 2012 and the Award of Excellence of the Austrian Ministry of Science in 2014. In the past years, he has led several data analysis groups in large projects with pharma industry. While Dr. Klambauer’s research has mainly been concerned with machine learning for molecular biology in earlier years, his research focus is currently on Deep Learning and Drug Discovery, concretely building AIs for automated drug discovery. This has led to the development of the best performing methods at the 2013 DREAM Toxicogenetics challenge and the win at the Tox21 Data Challenge 2015. He has published several impactful papers, such as "Deeptox: toxicity prediction using Deep Learning" introducing Deep Learning on molecules, and "Self-normalizing networks" crafting a novel type of neural networks. Günter Klambauer is currently leading the "AI in Drug Design" group of the LIT AI Lab at the Johannes Kepler University Linz.
Transforming Drug Design with High Content Imaging and Deep Learning
Large-scale assays for drug discovery are often too time- and cost-expensive although they are at the core of drug discovery and design. Therefore, the scientific community is searching for alternatives or to increase the throughput of these technologies. In this talk, we show how high content imaging data can be exploited to predict the biological activity of compounds and thus ameliorate the problem of large-scale assays.
Our proposed approach involves both image processing and machine learning techniques, of which multi-task deep learning is the best-performing method for this task of linking cell morphology and biological activity. Despite the high resolution and large data amount, we demonstrate that also convolutional neural networks can be used to predict assay outcomes in an end-to-end fashion. In two drug discovery projects, repurposing increased hit rates by 50- to 250-fold over that of the initial project assays while increasing the chemical structure diversity of the hits.Our results suggest that many biological assays could be replaced by high-content imaging together with Deep Learning methods.