Dragi Kocev
Jožef Stefan Institute, Slovenia
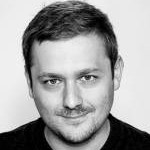
Biography: Dr. Dragi Kocev is a researcher at the Department of Knowledge Technologies, JSI. He completed his PhD in 2011 at the Jožef Stefan International Postgraduate School in Ljubljana on the topic of learning ensemble models for predicting structured outputs. He was a visiting research fellow at the University of Bari, Italy in 2014/2015. His research interests are in the field of data mining and includes the study, development and application of data mining algorithms; he is current research is aimed towards further development of efficient methods for learning from data with structured outputs (e.g., predicting multiple targets, hierarchical multi-label classification…) and their applications in machine vision, life sciences and ecological modelling. He has participated in several national Slovenian projects, the EU funded projects IQ and PHAGOSYS and is involved in the Human Brain Project. He was co-coordinator of the FP7 FET Open project MAESTRA.
Semi-supervised multi-target prediction for analysis of screening data
The predictive performance of traditional supervised methods heavily depends on the amount of labeled data. However, obtaining labels is a difficult process in many real-life tasks including compound screens, biomarker discovery etc. Only a small amount of labeled data is typically available for model learning. As an answer to this problem, the concept of semi-supervised learning has emerged. Semi-supervised methods use unlabeled data in addition to labeled data to improve the performance of supervised methods.
It is even more difficult to get labeled data for data mining problems with structured outputs since several labels need to be determined for each example. Multi-target prediction (MTP) is one type of a structured output prediction problem, where we need to simultaneously predict multiple variables. Despite the apparent need for semi-supervised methods able to deal with MTP, only a few such methods are available and even those are difficult to use in practice and/or their advantages over supervised methods for MTP are not clear.
We will present an algorithm for learning predictive models from limited amount of labelled data that can exploit the available unlabelled data in a way to yield models with better predictive performance. We will also show some benchmark experiments to assess their predictive performance. Finally, we will illustrate and discuss their use for analysis of high content screens.