Anna Cichonska
Department of Future Technologies, University of Turku
Institute for Molecular Medicine Finland FIMM, University of Helsinki
Department of Computer Science, Helsinki Institute for Information Technology HIIT, Aalto University, Finland
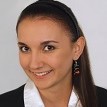
Biography: Anna Cichonska holds a PhD in machine learning in bioinformatics from Aalto University, Finland. Most of her research work has been conducted in collaboration with the Finnish Institute for Molecular Medicine FIMM. She has received the doctoral dissertation award 2019 from the School of Science of Aalto University. Her research is focused on the methods development for genome wide association studies as well as predictive modeling of molecular and phenotypic drug response signatures. Anna is one of the organizers of the IDG-DREAM Drug Kinase Binding Prediction Challenge which aims at evaluating the power of machine learning models as systematic and cost-effective means for catalyzing drug-protein interaction mapping efforts
Kernel-based predictive modelling of drug-protein binding affinities
Despite several years of target-based drug discovery, chemical agents inhibiting single targets are still rare. Mapping the complete protein target space of drugs and drug-like compounds, including both intended “primary targets” as well as secondary “off-targets”, is therefore a critical part of drug discovery efforts. Such a map would enable one not only to explore the therapeutic potential of chemical agents but also to better predict and manage their possible adverse effects prior to clinical trials. However, the massive size of the chemical universe makes experimental bioactivity mapping of the full space of compound-target interactions quickly infeasible in practice, even with the modern high-throughput profiling assays.
Machine learning methods provide a cost-effective and complementary approach to experimental drug bioactivity profiling, allowing for prioritization of the most potent drug candidates and protein target interactions for further verification in the laboratory. Recently, especially kernel-based methods have received significant attention in pharmacology offering, among others, the advantage of computationally efficient modelling of the nonlinearities between chemical and protein features and drug bioactivity profiles.
This lecture will introduce the concept of kernel learning and demonstrate how kernels can be used to model drug-protein interactions. We will focus on quantitative binding affinity prediction in order to fully characterize the activity spectrum of a drug. At the end of the lecture, we will also go through the summary of the results from the Illuminating the Druggable Genome (IDG)-DREAM Drug-Kinase Binding Prediction Challenge.