Adam Arany
KU Leuven, Belgium
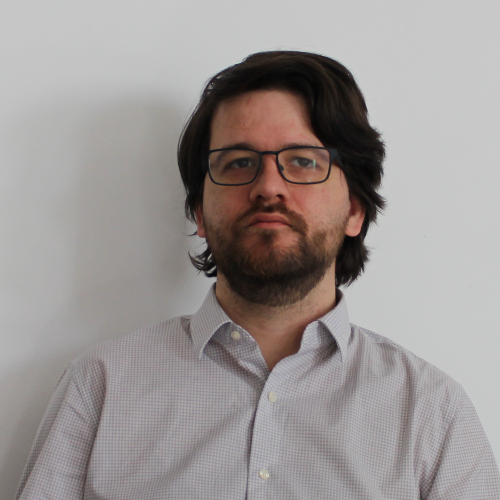
Biography: Adam Arany is currently a Postdoctoral Researcher at KU Leuven, Leuven, Belgium. His research interests are in the area of Chemoinformatics and Machine learning, especially Bayesian Methods and Deep Learning. He completed his MSc in Computer Science and Engineering at Budapest University of Technology and Economics, Budapest, Hungary. Then he completed his PhD studies in Pharmaceutical Chemistry at the Semmelweis University, Budapest, Hungary.
Multi-task learning in the analysis of phenotypic data
Multi-task learning is an efficient approach of machine learning which combines data from several related tasks, improving accuracy compared to solving each task separately. With the special requirements of large biological problems in mind, our research group developed a calable Bayesian matrix factorization method Macau, and its nonlinear deep learning based successor SparseFlow. In this talk I will illustrate the application of these methods in two application area related to phenotypic data analysis.
The first application is a proof-of-concept work demonstrating that data from a single high-throughput imaging assay can be repurposed to predict the biological activity of compounds in hundreds of assays targeting unrelated pathways or biological processes. Our results suggest that data from high-content screens are a rich source of information that can be used to predict and replace customized biological assays. These results also justify further work on image-based learning for drug discovery.
In the second application area we used the matrix factorization method to gain insight about the mechanisms underlying treatment efficacy in cancer, measured by the effect of cell type (gene expression) and drug targets to the effect of drugs on a given cell-line.